Learn about Benefits of AI Accelerators. Explore the benefits, types, and applications of AI accelerators. Discover how they revolutionize industries with speed, efficiency, and scalability, enabling groundbreaking technological advancements and AI-driven solutions.
Introduction to AI Accelerators
AI accelerators are hardware devices designed electronically to perform fast processing for Artificial intelligence tasks. Optimizing all computations for machine learning and deep learning algorithms speeds up the data handling processes and results in reduced energy consumption and efficient operation on complex workloads.
They are also commonly found in applications involving autonomous vehicles, healthcare, and retail applications. These AI accelerators are going to bring about revolutionary changes in industries with their unmatched speed and scalability potentials and fuel inventive transformation in a very game-changing technology domain.
What Are AI Accelerators?
AI accelerators can be specialized hardware or software that executes workloads based on AI more efficiently than conventional computing devices such as CPUs. These devices optimize tasks such as machine learning, deep learning, and data analysis by quickly handling mathematical operations. For instance, thousands of calculations and many chips and systems are required to train a neural network. With AI accelerators, one can speed up processing time and energy consumption.
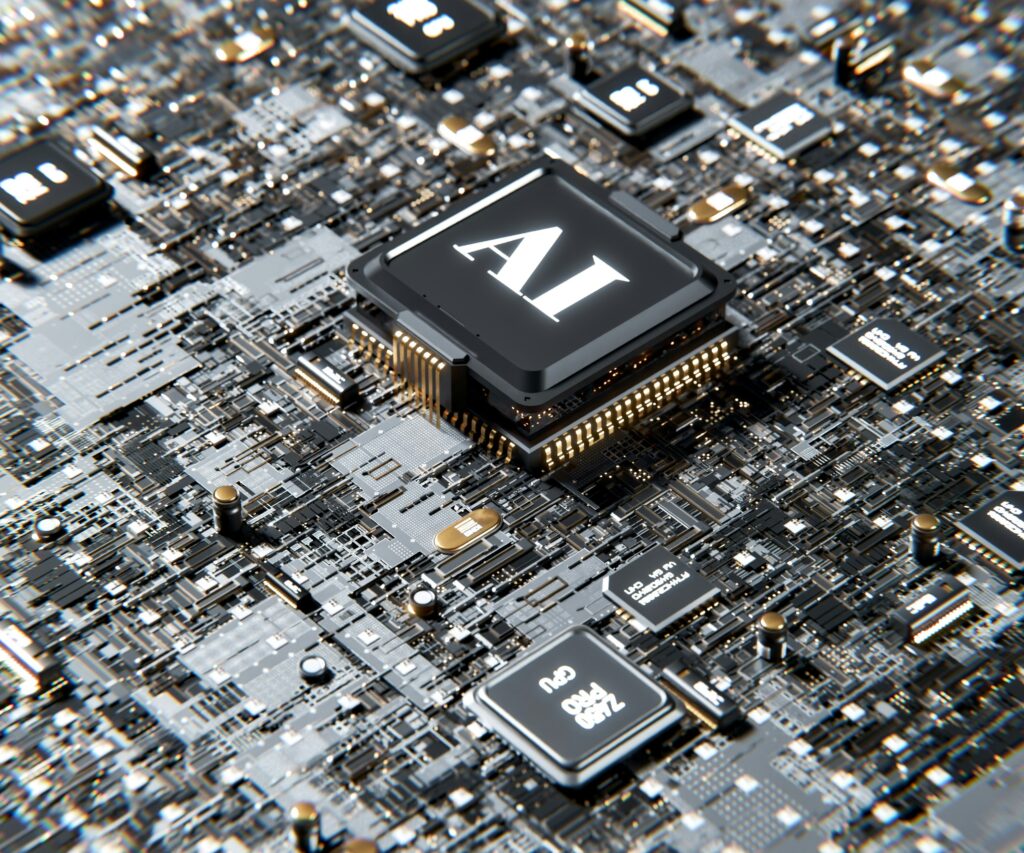
Types of AI Accelerators
1. GPUs (Graphics Processing Units)
GPUs are declarative accelerators that can parallel process any workload. Initially, they are optimized for high-speed graphics rendering. That is why they are the best devices for taking care of large-scale computations associated with AI tasks like deep learning or neural network training, making them the most popular among researchers and developers.
2. TPUs (Tensor Processing Units)
TPUs are developed by Google and are specialized for doing machine learning tasks – especially tensor computations – in a highly optimized way using deep learning frameworks. These chips are, therefore, appropriate for AI model training, inference, and performing these application workloads efficiently and optimally, such as object detection and presentation, as well as natural language processing.
3. FPGAs (Field-Programmable Gate Arrays)
FPGAs are application-flexed accelerators that can be programmed toward specific AI workloads. Their flexibility makes them apply to many broader uses, such as real-time data processing and edge computing, amidst trade-offs of performance and energy efficiency.
4. ASICs (Application-Specific Integrated Circuits)
ASICs are designed for one specific task under the hood, hence run most efficiently and fastest in every processing stage. That’s why they are becoming increasingly important in applications where the highest possible demand, such as autonomous cars and huge-scale AI data centers, can exploit high performance at very low energy consumption of dedicated workloads.
Benefits of AI Accelerators
1. Speed
AI accelerators process huge amounts of data much faster than traditional processors. They optimize parallel computing tasks to execute machine learning models more quickly, allowing real-time operations and reducing delays in critical systems such as autonomous vehicles and medical diagnostics.
2. Efficiency
AI accelerators comprise several computing cores: CPUs, GPUs, and specialized processors. Diverse types of workloads can, thus, be handled, including training and inference. It also has an open ability to refine and deliver optimum performance for more advanced AI-related tasks, such as deep learning, data analysis, and natural language processing.
3. Heterogeneous Architecture
AI accelerators integrate various computing cores, combining CPUs, GPUs, and specialized processors. This heterogeneous design supports diverse workloads, including training and inference, providing flexibility and performance for advanced AI tasks like deep learning, data analysis, and natural language processing.
4. Cost-effectiveness
Operational costs for AI computations are reduced, their leading efficiencies rendering AI computations possible and affordable. In high performance with reduced power and maintenance costs, the hardware and software optimization route makes AI viable for businesses, small or large in number.
5. Energy-efficient Edge Devices
AI accelerators provide intelligence to an edge device at minimal energy consumption. This enables real-time processing and additional functionalities while increasing battery life in wearable technology, smart home systems, and remote sensors-something that IoT devices should have.
6. Scalability
In modular architectures, AI accelerators can easily scale up by the time workloads grow. Adapting to changes in AI models is possible; thus companies can enhance their AI potential without overhauling infrastructure, an application that suits the roving environment such as e-commerce and healthcare.
7. Improved Productivity and Efficiency
Automation of complex processes together with improved data-based decisions by AI accelerators are beneficial to productivity. Such work, reduced human errors and improved efficiencies make possible the operational excellence of industries in logistics, manufacturing, and customer service.
Use of AI Accelerators
1. Autonomous Vehicles
AI accelerators empower self-driving technology to process data in real time for navigation, obstacle detection, and decision-making. Sophisticated algorithms for adaptive cruise control, lane detection, or even emergency braking systems ensure they drive these powerful puppies safely in a flash.
2. Retail
Retailers could use AI accelerators for personalized shopping encounters, demand forecasts, and stock management. These gadgets could analyze the customer’s tastes in real time, product recommendations, or continuously adapt processes to ensure increased customer satisfaction and sales.
3. Chatbots
AI accelerators take a step forward in enriching chatbots through rapid natural language processing. Bots understand, comprehend, and respond to customer queries accurately and quickly through AI accelerators, facilitating smooth end-user experience in applications, such as customer support, e-commerce, or banking.
4. Healthcare
AI accelerators process medical images and predict patient outcomes, besides tailoring treatment plans. It helps bring advancements in drug discovery, diagnosis of disease, and telemedicine, which directly increases the quality of patient care.
5. Real Estate
Property values can be calculated, tours can be taken virtually, and market trends are analyzed by AI accelerators in the real estate sector. Such processing of huge datasets would enable buyers, sellers, and agents to arrive at decisions fairly quickly and efficiently.
6. Higher Education
This enables adaptive learning venues, individualized student experience, and AI research in education. AI accelerators process intricate algorithms efficiently, thereby generating innovations as well as improved teaching methodologies in educational institutions.
Why Do We Need AI Accelerators?
Today, it is practically impossible for traditional computing infrastructure to face the explosion of AI applications such as virtual assistants and driverless cars. Here is where AI accelerators fill the gap:
- Faster data processing
- Better handling of large datasets
- High efficiency in power consumption for intensive computations
AI accelerators form the backbone of today’s AI systems because they provide scalability and reliability.
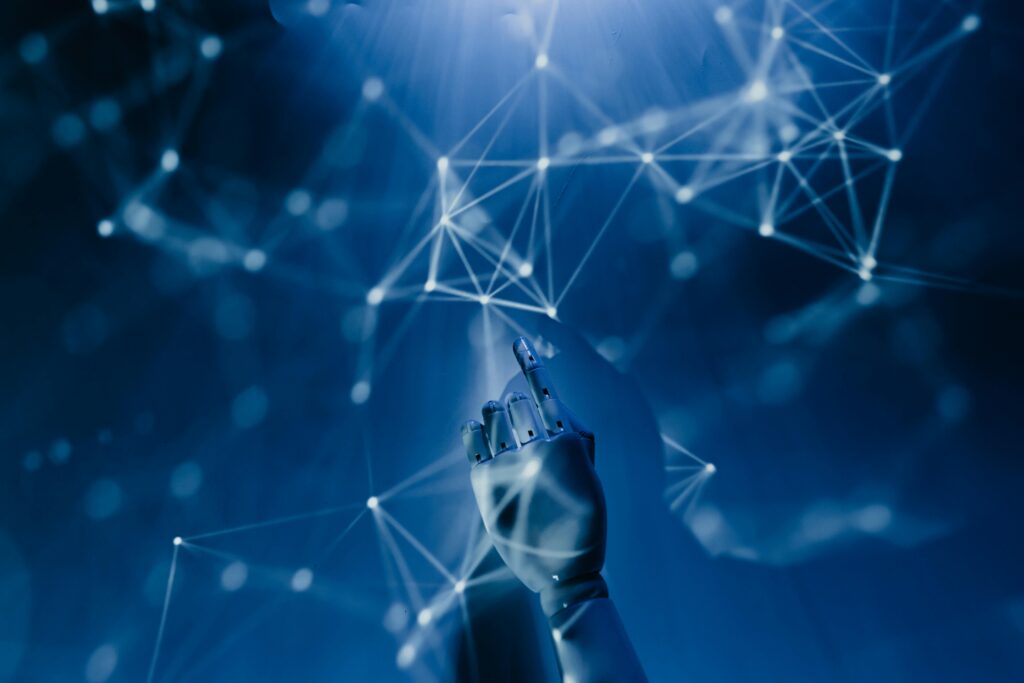
How AI Accelerators Work
1. Specialized Hardware
Hardware engines, which are called AI accelerators, differ from general-purpose processors because they have optimized hardware for specific tasks like neural network calculations. These speedier programs are energy-efficient and expect complex workloads as they are highly specialized.
2. Parallel Computing
They process several computations at once. Thus, performance improves considerably when undertaking activities such as training models for machine learning or performing inferences for AI-based systems.
3. Low Precision Arithmetic
AI calculators operate using low-precision arithmetic by completing calculations with narrow bit-widths. This enhances speed while reducing power consumption, but it is still within an acceptable limit of accuracy for activities like image recognition or language processing.
4. Tailored Memory Hierarchies
Tailored memory architectures such as these provide faster access to data with minimum bottlenecks. Using AI workloads to match memory systems achieves processing efficiencies in heavy resource consumption applications.
5. Heterogeneous Architecture
AI accelerators pack heterogeneous processing cores such as CPUs, GPUs, and dedicated functional units on a single architecture, suitable for a wide variety of AI applications training to inference, thus enhancing versatility.
6. Integrated Photonics
Some sophisticated AI accelerators use integrated photonics: transmitting light rather than electrical signals for data transfer. This technology increases the speed and energy efficiency of the devices, especially in high-performance computing environments.
FAQs
How do AI accelerators differ from traditional CPUs?
AI accelerators are indeed experts in parallel processing and mathematics, which makes them far more efficient than general-purpose CPUs for the workloads in AI.
Can AI accelerators be used for non-AI tasks?
The GPUs are mainly designed for AI work, but they can also be used to do something other than AI, for instance, video rendering or havoc operations.
Are AI accelerators environmentally friendly?
Although AI accelerators are energy efficient compared to conventional processors, they are still energy-consuming in manufacturing and operating.
What is the role of AI accelerators in cloud computing?
Cloud AI accelerators enable scalable high-performance computing on demand for AI applications without requiring organizations to put in thousands or millions of dollars on hardware.
Conclusion
AI accelerators are the backbone of modern advancements in AI, starting from virtual assistants going all the way to autonomous cars. It is speed, efficiency, and scalability that transform industries and extend the boundaries of what’s possible in technology. However, high costs and ethical issues need to be addressed before we fully embrace the way towards this transformation. AI accelerators have a bright future ahead; they promise new dimensions of innovation and discovery.